Seven papers by CSE researchers presented at ACL 2023
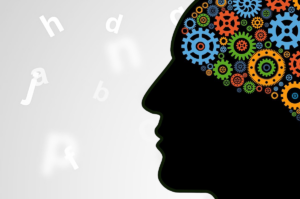
A total of seven papers by CSE researchers have been accepted for presentation at the 61st Annual Meeting of the Association for Computational Linguistics (ACL), taking place place July 9-14 in Toronto, Canada. The ACL is the premier global society for researchers focused on computational linguistics and natural language processing.
The research being presented by CSE-affiliated authors at ACL 2023 covers a breadth of topics in these areas, including grounded vocabulary acquisition in vision-language models, analogical reasoning in pre-trained language models, tunable text generation, and more. These papers are listed below, with the names of CSE researchers in bold:
Human Inspired Progressive Alignment and Comparative Learning for Grounded Word Acquisition
Yuwei Bao, Barrett Lattimer, and Joyce Chai
Abstract: Human language acquisition is an efficient, supervised, and continual process. In this work, we took inspiration from how human babies acquire their first language, and developed a computational process for word acquisition through comparative learning. Motivated by cognitive findings, we generated a small dataset that enables the computation models to compare the similarities and differences of various attributes, learn to filter out and extract the common information for each shared linguistic label. We frame the acquisition of words as not only the information filtration process, but also as representation-symbol mapping. This procedure does not involve a fixed vocabulary size, nor a discriminative objective, and allows the models to continually learn more concepts efficiently. Our results in controlled experiments have shown the potential of this approach for efficient continual learning of grounded words.
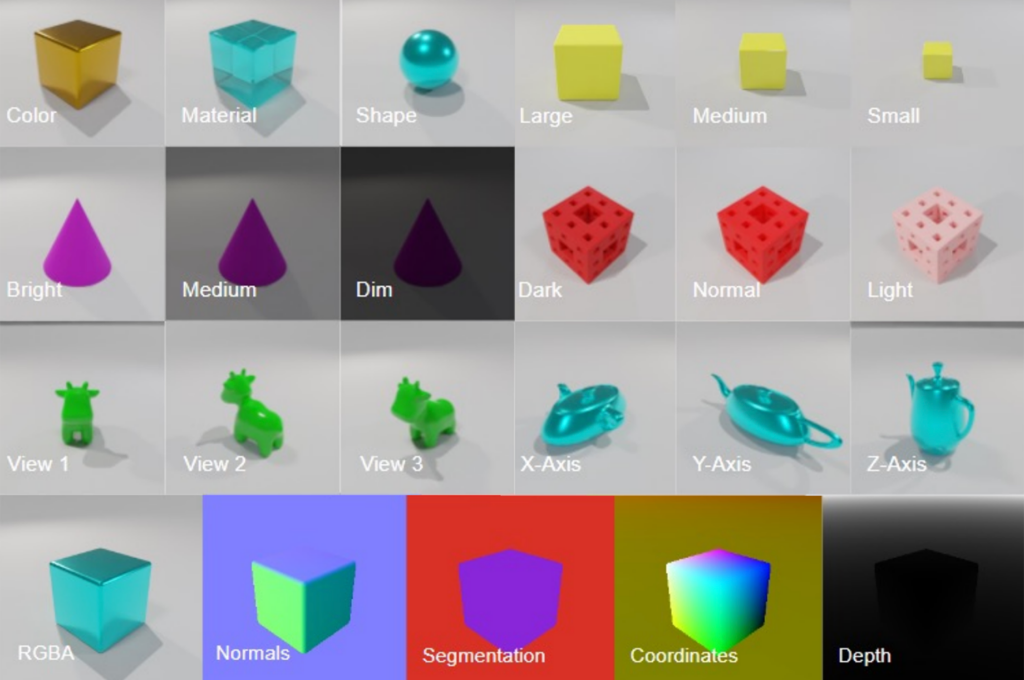
In-Context Analogical Reasoning with Pre-Trained Language Models
Xiaoyang Hu, Shane Storks, Richard Lewis, and Joyce Chai
Abstract: Analogical reasoning is a fundamental capacity of human cognition that allows us to reason abstractly about novel situations by relating them to past experiences. While it is thought to be essential for robust reasoning in AI systems, conventional approaches require significant training and/or hard-coding of domain knowledge to be applied to benchmark tasks. Inspired by cognitive science research that has found connections between human language and analogy-making, we explore the use of intuitive language-based abstractions to support analogy in AI systems. Specifically, we apply large pre-trained language models (PLMs) to visual Raven’s Progressive Matrices (RPM), a common relational reasoning test. By simply encoding the perceptual features of the problem into language form, we find that PLMs exhibit a striking capacity for zero-shot relational reasoning, exceeding human performance and nearing supervised vision-based methods. We explore different encodings that vary the level of abstraction over task features, finding that higher-level abstractions further strengthen PLMs’ analogical reasoning. Our detailed analysis reveals insights on the role of model complexity, in-context learning, and prior knowledge in solving RPM tasks.
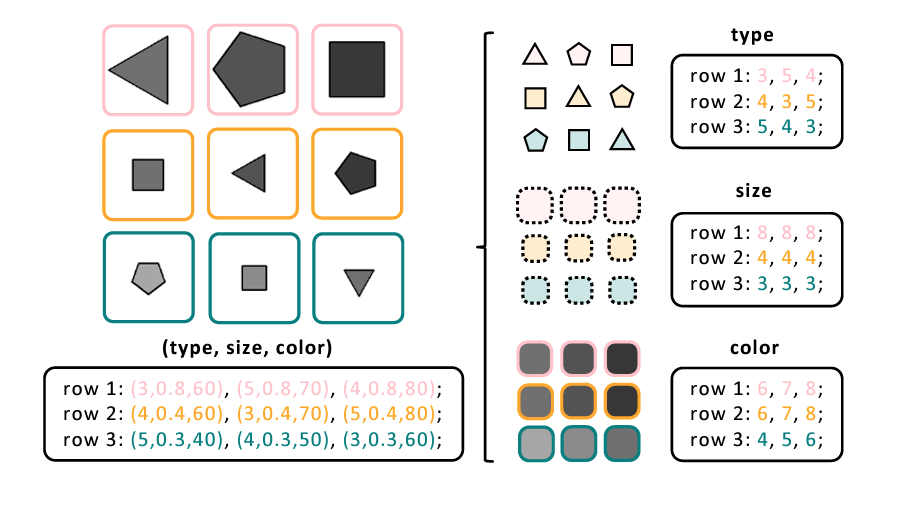
World-to-Words: Grounded Open Vocabulary Acquisition through Fast Mapping in Vision-Language Models
Ziqiao Ma, Jiayi Pan, and Joyce Chai
Abstract: The ability to connect language units to their referents in the physical world, referred to as grounding, is crucial to learning and understanding grounded meanings of words. While humans demonstrate fast mapping in new word learning, it remains unclear whether modern vision-language models can truly represent language with their grounded meanings and how grounding may further bootstrap new word learning. To this end, we introduce Grounded Open Vocabulary Acquisition (GOVA) to examine grounding and bootstrapping in open-world language learning. As an initial attempt, we propose object-oriented BERT (OctoBERT), a novel visually-grounded language model by pre-training on image-text pairs highlighting grounding as an objective. Through extensive experiments and analysis, we demonstrate that OctoBERT is a more coherent and fast grounded word learner, and that the grounding ability acquired during pre-training helps the model to learn unseen words more rapidly and robustly.
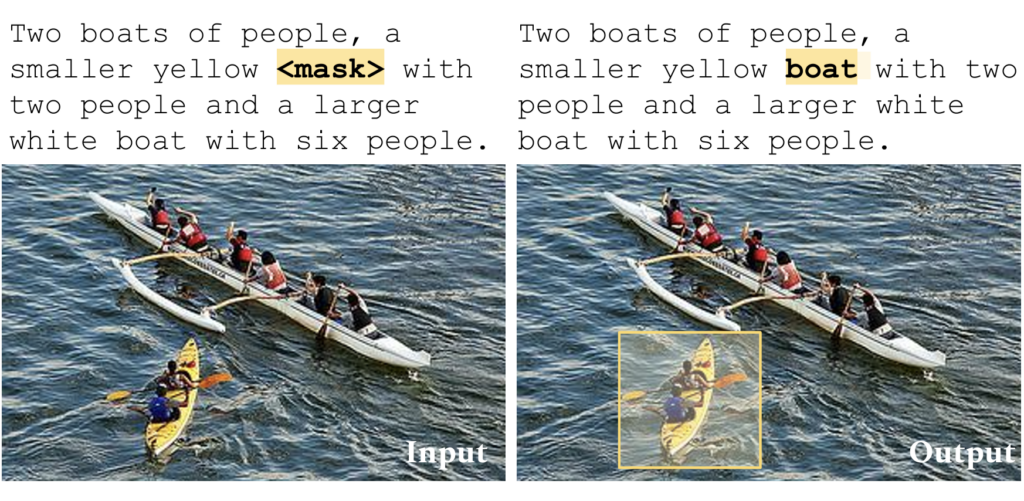
NLP Reproducibility For All: Understanding Experiences of Beginners
Shane Storks, Keunwoo Yu, Ziqiao Ma, and Joyce Chai
Abstract: As natural language processing (NLP) has recently seen an unprecedented level of excitement, and more people are eager to enter the field, it is unclear whether current research reproducibility efforts are sufficient for this group of beginners to apply the latest developments. To understand their needs, we conducted a study with 93 students in an introductory NLP course, where students reproduced the results of recent NLP papers. Surprisingly, we find that their programming skill and comprehension of research papers have a limited impact on their effort spent completing the exercise. Instead, we find accessibility efforts by research authors to be the key to success, including complete documentation, better coding practice, and easier access to data files. Going forward, we recommend that NLP researchers pay close attention to these simple aspects of open-sourcing their work, and use insights from beginners’ feedback to provide actionable ideas on how to better support them.
BOLT: Fast Energy-based Controlled Text Generation with Tunable Biases
Xin Liu, Muhammad Khalifa, and Lu Wang
Abstract: Energy-based models (EBMs) have gained popularity for controlled text generation due to their high applicability to a wide range of constraints. However, sampling from EBMs is non-trivial, as it often requires a large number of iterations to converge to plausible text, which slows down the decoding process and makes it less practical for real-world applications. In this work, we propose BOLT, which relies on tunable biases to directly adjust the language model’s output logits. Unlike prior work, BOLT maintains the generator’s autoregressive nature to assert a strong control on token-wise conditional dependencies and overall fluency, and thus converges faster. When compared with state-of-the-arts on controlled generation tasks using both soft constraints (e.g., sentiment control) and hard constraints (e.g., keyword-guided topic control), BOLT demonstrates significantly improved efficiency and fluency. On sentiment control, BOLT is 7x faster than competitive baselines, and more fluent in 74.4% of the evaluation samples according to human judges.
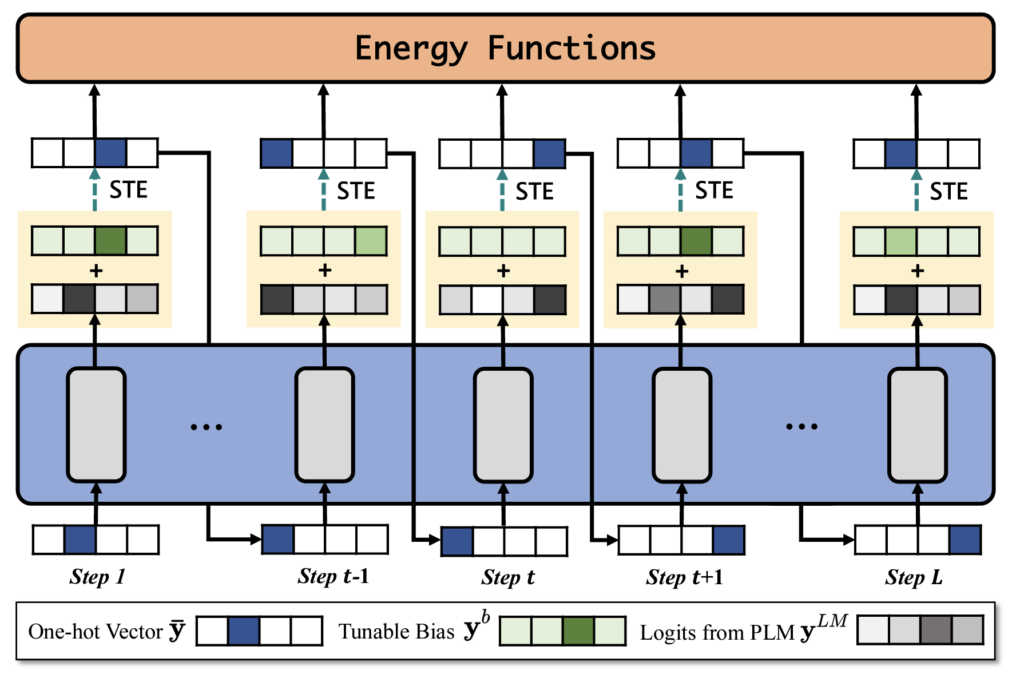
Few-shot Reranking for Multi-hop QA via Language Model Prompting
Muhammad Khalifa, Lajanugen Logeswaran, Moontae Lee, Honglak Lee, and Lu Wang
Abstract: We study few-shot reranking for multi-hop QA (MQA) with open-domain questions. To alleviate the need for a large number of labeled question-document pairs for retriever training, we propose PromptRank, which relies on large language models prompting for multi-hop path reranking. PromptRank first constructs an instruction-based prompt that includes a candidate document path and then computes the relevance score between a given question and the path based on the conditional likelihood of the question given the path prompt according to a language model. PromptRank yields strong retrieval performance on HotpotQA with only 128 training examples compared to state-of-the-art methods trained on thousands of examples — 73.6 recall@10 by PromptRank vs. 77.8 by PathRetriever and 77.5 by multi-hop dense retrieval.
Rule By Example: Harnessing Logical Rules for Explainable Hate Speech Detection
Christopher Clarke, Matthew Hall, Gaurav Mittal, Ye Yu, Sandra Sajeev, Jason Mars, and Mei Chen
Abstract: Classic approaches to content moderation typically apply a rule-based heuristic approach to flag content. While rules are easily customizable and intuitive for humans to interpret, they are inherently fragile and lack the flexibility or robustness needed to moderate the vast amount of undesirable content found online today. Recent advances in deep learning have demonstrated the promise of using highly effective deep neural models to overcome these challenges. However, despite the improved performance, these data-driven models lack transparency and explainability, often leading to mistrust from everyday users and a lack of adoption by many platforms. In this paper, we present Rule By Example (RBE): a novel exemplar-based contrastive learning approach for learning from logical rules for the task of textual content moderation. RBE is capable of providing rule-grounded predictions, allowing for more explainable and customizable predictions compared to typical deep learning-based approaches. We demonstrate that our approach is capable of learning rich rule embedding representations using only a few data examples. Experimental results on 3 popular hate speech classification datasets show that RBE is able to outperform state-of-the-art deep learning classifiers as well as the use of rules in both supervised and unsupervised settings while providing explainable model predictions via rule-grounding.