Women in Computing
Combining Human and Machine Intelligence to Tackle Social Science Problems
Add to Google Calendar
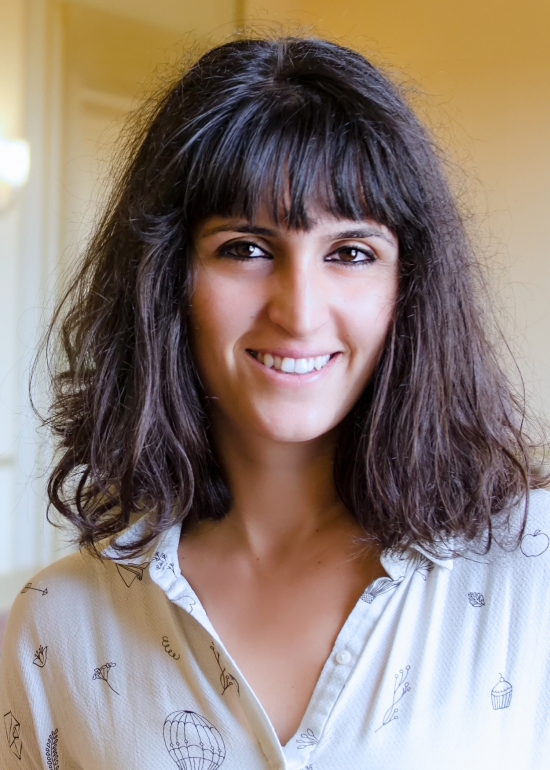
Combining the complementary strengths of human and machine intelligence has the potential to shed new light on old questions in the social sciences. In this talk, I will demonstrate this potential by focusing on the applications of crowdsourcing and large scale content analysis techniques to two different problems. I will first present a recent work that investigates the selection and framing of political news at scale. Our study employs supervised learning methods to identify articles pertaining to political events in a large news corpus and next recruits online human judges to classify a representative sample of political articles according to topic and ideological position in a large randomized experiment. Such an analysis not only provides a reliable index of media bias but also allows us to investigate how this bias varies across different issues. Next, I will briefly discuss how human and machine intelligence can be employed to characterize charitable giving behavior and estimate the impact a widely adopted recommendation system could have in the charity marketplace.
Ceren Budak is an Assistant Professor at the University of Michigan School of Information. Her research interests lie in the area of computational social science; a discipline at the intersection of computer science, statistics, and the social sciences. She is particularly interested in applying large scale data analysis techniques to study problems with social, political, and policy implications.