Dissertation Defense
Enforcing Realism and Temporal Consistency for Large-Scale Video Inpainting
This event is free and open to the publicAdd to Google Calendar
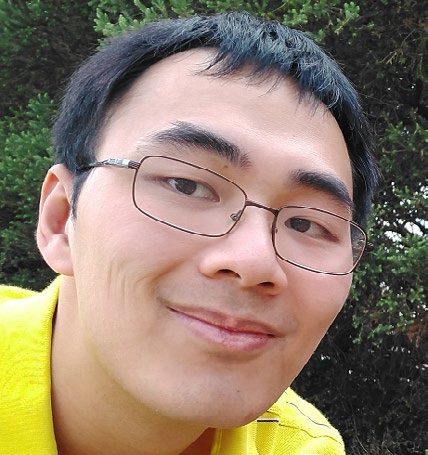
Virtual dissertation defense (Passcode: 758561)
ABSTRACT: Video manipulation is rapidly gaining traction due to the influence of viral videos and the convenience of editing software. Although it has legitimate entertainment purposes, it can also be incredibly destructive. To understand the positive and negative consequences of video manipulation, it is important to investigate the limits of its capabilities.
In this dissertation, we focus on the advanced manipulation task of video inpainting, whose goal is to automatically fill in missing parts of a masked video with semantically relevant content. Prior work has struggled with semantic ambiguity—which arises from several plausible explanations of events in the observed scene—due to their exploitation of limited temporal contexts. They have also underemphasized fine-grained analysis of inpainting failure modes; as a result, the behaviors of models under specific scenarios have remained poorly understood. Our work improves on both models and evaluation techniques for video inpainting, thereby providing deeper insight into how an inpainting model’s design impacts the visual quality of its outputs.
We propose two models, bi-TAI and HyperCon, that improve visual quality by expanding the available temporal context. bi-TAI inpaints full, consecutive frames by intelligently integrating information from multiple frames before and after the desired sequence; HyperCon suppresses flickering artifacts from frame-wise processing by identifying and propagating consistencies found in high frame rate space.
We also propose two evaluation tools to diagnose failure modes of modern video inpainting methods. We use our Moving Symbols dataset to characterize the sensitivity of a video prediction model to controllable appearance and motion parameters. Meanwhile, our DEVIL benchmark provides a dataset and comprehensive evaluation scheme to quantify how semantic properties of videos and masks affect inpainting quality. Through models that exploit expanded temporal contexts—as well as evaluation paradigms that reveal fine-grained failure modes of inpainting methods at scale—we enforce better visual quality for video inpainting on a larger scale than prior work.